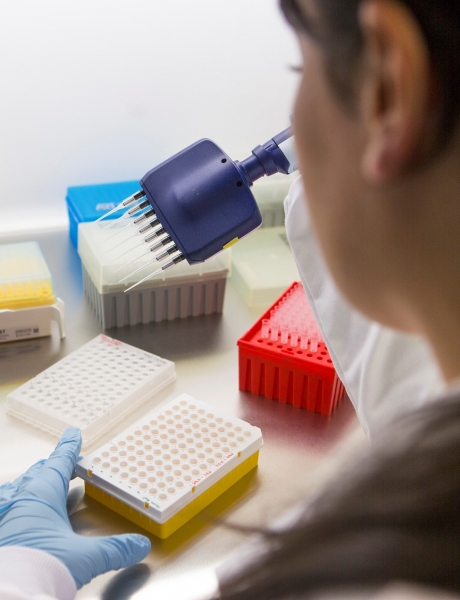
The development of computational models to predict drug response and enhance understanding of cancer onset and progression based on an individual’s molecular profile, is providing new opportunities for personalised medicine in oncology. Computational models based on ordinary differential equations (ODEs) are among the most promising approaches for quantitatively capturing the dynamic behavior of the complex cellular processes associated with cancer and facilitating individualised predictions of drug response.
Models are converted into systems of ODEs, which can be solved numerically, if (and only if) we assume values for the parameters (e.g. binding affinities, (de)phosphorylation rates, synthesis and degradation rates) of these equations. A key challenge for truly personalised tumour therapy is therefore to identify those regions of the computational model parameter space that lead to the most accurate predictions.
Recent proof-of-principle analyses from our consortium are showing the potential of novel orders of magnitude more efficient experimental and computational parameter optimisation strategies for overcoming this hurdle. With a focus on triple negative breast cancer (TNBC), a tumour entity with a high socio-economic impact and high unmet medical need, PeCaN will combine Artificial Intelligence, single-cell transcriptomics/proteomics and CRISPR-based cell genetics to parameterise a large-scale computational model for personalising therapy choice in individual patients, and to identify new drug targets and biomarkers. Candidates and predictions will be evaluated clinically, using patients from multiple clinical sites to assess the new clinical decision support system, and to initiate future prospective trials for TNBC, validating relevant biomarker/drug combinations. To support the clinical translation of the proposed model-based approach, an in-depth Health Technology Assessment will be carried out, covering normative, regulatory and economic aspects.